Artificial Intelligence
A Comprehensive Insight into Large Language Models: Concepts, Applications, and Development Tools
Artificial Intelligence (AI) has become an integral part of our digital lives and at the heart of this technological marvel are Large Language Models (LLMs). These colossal databases of textual information empower AI applications to perform tasks that seem almost human-like, ranging from engaging in conversation to generating art or analyzing complex data sets.
LLMs have a wide array of applications, including chatbots for enhanced customer interactions, sentiment analysis to gather feedback and improve services, content moderation on social media platforms, advanced translation services, efficient email filtering, writing and editorial assistance, and coding and software development. These models come in various types, each tailored for specific applications, such as natural conversation models, emotion analysis, multilingual translation models, content moderation, and spam detection.
The development process for AI applications has been simplified by low-code and no-code tools like Chainlit, LLMStack, FlowiseAI, Stack AI, and Voiceflow. These tools offer visual editors, drag-and-drop interfaces, and pre-built components, making it easier for developers and non-developers alike to integrate LLMs into their applications.
The article concludes with a practical demonstration of building an AI career assistant using FlowiseAI. This assistant, trained with LLMs, offers personalized career advice by analyzing user inputs like interests, skills, and career aspirations. The development process involves setting up retrievers, creating a multi-prompt chain, integrating language models, adding a conversational agent, incorporating web search capabilities, building in memory, and assembling the final workflow. This demonstration illustrates the interconnectedness of various components within an AI application and their collective role in creating an intelligent and responsive AI assistant.
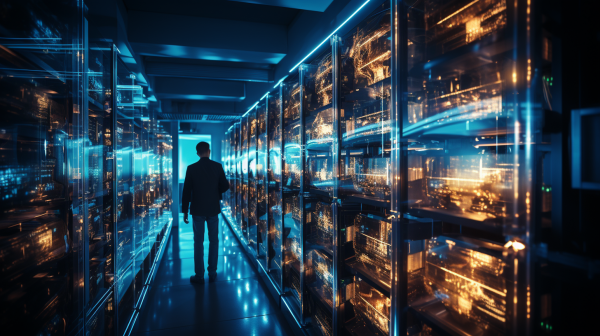
Artificial Intelligence (AI), often perceived as a complex and enigmatic field, is increasingly becoming integral to our digital lives. At the heart of this technological marvel are Large Language Models (LLMs), which empower AI applications to perform tasks that seem almost human-like in their execution. This article delves into the essence of LLMs, explores their diverse use cases, and examines a range of tools designed to simplify their integration into various applications.
Understanding Large Language Models
LLMs are essentially colossal databases of textual information. They are the cornerstone of AI systems, allowing these systems to utilize the extensive language understanding developed during their training. Imagine infusing a digital brain with a vast array of knowledge and then deploying it to perform specific tasks – this is the role of an LLM in AI.
The concept of “knowledge” in AI is layered and multifaceted. Just like humans can be “book smart” or “street smart,” LLMs can be trained with different types of data to be versatile in various contexts. Whether it’s engaging in conversation, generating art, or analyzing complex data sets, the AI’s capability depends on the nature and scope of the data it has been trained on.
Expanding Use Cases of LLMs
LLMs have a wide array of applications, which are continually evolving. Some of the prominent use cases include:
- Chatbots for Enhanced Customer Interactions: Companies like Salesforce have pioneered the use of LLMs in creating responsive chatbots for customer support and engagement.
- Sentiment Analysis: LLMs, like Grammarly’s tone detector, analyze text to assess emotions, helping businesses gather feedback and improve their services.
- Content Moderation: LLMs aid in maintaining community standards on social media platforms by identifying and filtering inappropriate content.
- Advanced Translation Services: Innovations like Meta AI’s SeamlessM4T represent significant advancements in speech-to-speech and text-to-text translation, showcasing the potential of LLMs in breaking language barriers.
- Efficient Email Filtering: LLMs are instrumental in distinguishing between legitimate emails and spam, a technology utilized by companies like Google to enhance user experience.
- Writing and Editorial Assistance: Tools like Grammarly and Hemingway use LLMs to offer writing suggestions, enhancing the overall quality of written content.
- Coding and Software Development: LLMs have made a foray into the development sector with tools like GitHub Copilot and Amazon’s CodeWhisperer, aiding in code completion and quality enhancement.
Types of Models and Their Applications
The diversity of LLMs is vast, each tailored for specific applications:
- Natural Conversation Models: LLMs like Anthropic’s Claude are designed for engaging conversations, making them ideal for chatbots and virtual assistants.
- Emotion Analysis: Models like Falcon are tuned for sentiment analysis, offering a nuanced understanding of emotional content in text.
- Multilingual Translation Models: SeamlessM4T by Meta AI is a prime example of a multilingual model enabling real-time translation across languages.
- Content Moderation: OpenAI’s API includes LLMs specifically trained for identifying and flagging toxic content.
- Spam Detection: Certain LLMs specialize in text classification tasks, such as detecting spam in emails.
Beyond Large Models
Not all language models are “large.” Smaller models, tailored for specific or niche tasks, offer personalized experiences without the extensive knowledge base of larger counterparts. These models, like the one used by Luke Wrobleski on his website, provide responses that are more targeted and context-specific.
Low-Code Tools for LLM Integration
With the growing complexity of AI technologies, low-code, and no-code tools have emerged to democratize access to LLM integration. These tools simplify the development process, making AI more accessible to a broader audience. Some notable platforms include:
- Chainlit: An open-source Python package offering a visual editor for building ChatGPT-style interfaces.
- LLMStack: A versatile platform for creating AI apps and chatbots, allowing data to be channeled through various models.
- FlowiseAI: Known for its drag-and-drop interface, this tool simplifies the process of connecting apps with LLM APIs.
- Stack AI: A no-code platform featuring a range of LLM offerings and data loaders for integrating with various data sources.
- Voiceflow: Specialized in developing voice assistant and chat applications, ideal for niche voice-based AI projects.
Practical Demonstration: AI Career Assistant with FlowiseAI
To illustrate the practical application of these concepts, let’s consider developing an AI-powered career assistant using FlowiseAI. This assistant, trained with LLMs, offers personalized career advice by analyzing user inputs like interests, skills, and career aspirations. It leverages various components such as retrievers, chains, memory, and conversational agents to provide
This assistant utilizes multiple components such as retrievers, chains, language models, memory, and conversational agents, offering a hands-on example of how these elements interact within an AI application.
Setting Up the Workflow
The development process begins with the setup of retrievers. These are essentially templates that the multi-prompt chain queries. Different retrievers fetch various types of information, like documents or data, which are then used to form the responses of the AI assistant.
In the FlowiseAI interface, we first add a Prompt Retriever to our project. This is a crucial step, as the Prompt Retriever serves as the gateway to obtain necessary information. For our career assistant, we configure it to suggest careers, recommend tools, provide salary information, and identify suitable locations.
Creating a Multi-Prompt Chain
A Multi-Prompt Chain allows us to establish a conversational interaction between the user and the AI assistant. By combining the prompts we’ve added to the canvas and connecting them to appropriate tools and language models, we enable the assistant to prompt users for information and process their responses to generate career advice.
Integrating Language Models
For our demonstration, we integrate Anthropic’s Claude, a versatile LLM designed for complex reasoning and creative tasks. This model is connected to the Multi-Prompt Chain, allowing the AI assistant to leverage Claude’s capabilities in generating responses.
Adding a Conversational Agent
The next step involves integrating a Conversational Agent. This component enables the AI assistant to perform a range of tasks, such as accessing the internet or sending emails. It acts as a bridge connecting external services and APIs, thus enhancing the versatility of the assistant.
Incorporating Web Search Capabilities
To enable the AI assistant to perform web searches for gathering information, we integrate tools like the Serp API. After configuring it with the necessary API keys, we connect it to the Conversational Agent, thus allowing the assistant to perform bespoke web searches as part of its functionality.
Building In Memory
The Memory component is vital as it allows the AI assistant to retain information from conversations. This feature is crucial for referencing past interactions and ensuring a coherent and context-aware dialogue with users. We add the Buffer Memory node to our project, which stores the raw input of past conversations for future reference.
The Final Workflow
Our final workflow comprises several interconnected components:
- Prompt Retrievers: These provide the templates for the AI assistant to converse with the user.
- Multi-Prompt Chain: It connects the prompt retrievers and selects the appropriate tools and language models based on user interaction.
- Claude Language Model: It is linked to the multi-chain prompt, providing intelligence for generating responses.
- Conversational Agent: This agent, connected to the Claude model, allows the assistant to perform additional tasks, such as Google searches.
- Serp API: It is connected to the conversational agent for conducting specific web searches.
- Buffer Memory: This component, linked to the conversational agent, stores conversation history.
This comprehensive setup in FlowiseAI provides a detailed visualization of how an AI application operates, illustrating the interconnectedness of its various components and their collective role in creating an intelligent and responsive AI assistant.
Conclusion
Through this detailed demonstration with FlowiseAI, we have peeled back the layers of the AI “black box,” revealing the intricate workings of LLMs and their integration into AI applications. From chatbots to translation services, and now to personalized career advice, the capabilities of LLMs are vast and ever-expanding.
As AI continues to evolve and integrate into more aspects of our lives, understanding and harnessing the power of LLMs becomes increasingly important. Whether you are a developer, a marketer, or just an AI enthusiast, the potential applications of these models are limited only by the imagination. What new innovations and applications will you create using the power of Large Language Models?
Marketing
101 of the best blogging tools in 2024,
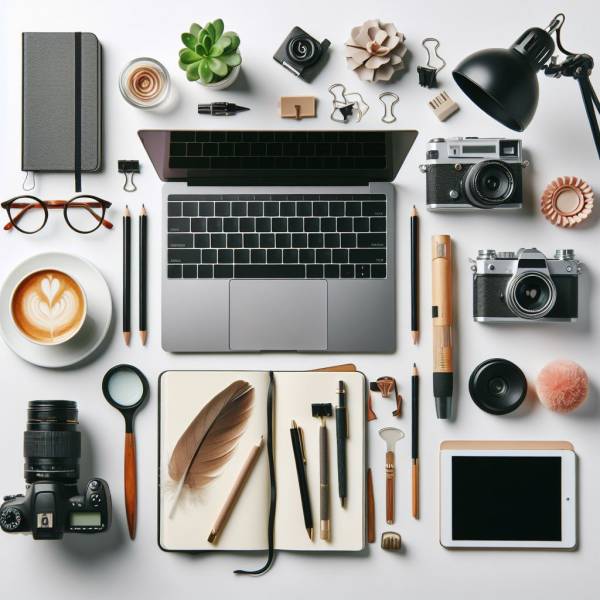
101 of the best blogging tools in 2024.
Having the best blogging tools continues to be critical for bloggers in 2024. In this comprehensive updated list for 2024, I’ve ranked just some of the most impactful free and paid blogging tools you should prioritize to build, manage, and grow your blog this year in no particular order.
We cover the latest developments in marketing, AI writing assistants, research, SEO, social media, design, editing, and more; having the right tools from day one – and knowing when to advance to more powerful options as your blog scales – is imperative.
These blogging tools save time and effort and allow you to analyze performance better, tap into new audiences, and grow revenue streams that wouldn’t exist without the proper technology stack powering a modern blog.
AI Tools to Enhance Blogging
Artificial intelligence has introduced phenomenal advancements in content creation, idea generation, editing, and graphics. As bloggers, these AI tools present incredible opportunities to improve our workflows, ideation, writing, and visual media.
The latest advancements in 2024 even allow AI tools to produce high-quality, engaging articles that capture your blog’s voice. Here, I’ll overview my top recommendations across categories that any blogger can test out today to supercharge their efforts:
1. Ahrefs
Ahrefs, while more advanced, has cemented itself as an indispensable SEO and content research juggernaut. It goes far beyond just tracking keyword rankings and backlinks with tools for content research, topic exploration, competitor analysis, technical audits, and more.
I often use Features like Content Explorer to uncover trending topics and gaps in my niche. Competitor Research quickly compares my performance against other sites for strategic keywords. And with the largest backlink database around, no other platform lets me analyze backlinks as deeply as Ahrefs.
While newer bloggers may find it information-dense, Ahrefs packs immense value if you take the time to master it. Many consider it well worth the monthly investment. Those wanting a simpler alternative should check out Semrush.
2. MonsterInsights
This popular WordPress plugin seamlessly connects Google Analytics with your website. Without any coding, MonsterInsights adds enhanced tracking for site searches, outbound links, downloaded files, and custom events.
Seeing your analytics directly within the WordPress dashboard is a huge time-saver. MonsterInsights also lets you test different content layouts and headlines with its built-in A/B testing tool. I consider it an essential free install for bloggers wanting deeper insight from Google Analytics.

3. Serp Robot
Serp Robot is my go-to free rank tracker, which monitors your keyword positions across search engines. Unlike other rank checkers, Serp Robot is shockingly accurate at picking up the many fluctuations in rankings week-to-week.
Sudden position drops could hint at penalties, making ongoing rank tracking all the more important. For simplicity and reliability, Serp Robot is a great basic addition to stay updated on how you rank for target keywords.
4. Semrush
SEMrush has exploded into a dominant marketing suite over the past few years. While a tad pricier than alternatives, you get unmatched bang for your buck with over 100 integrated tools.
I regularly use SEMrush for keyword research, tracking ranking progress, analyzing backlinks, monitoring brand mentions, exploring related keywords, spying on the competition, and more. It handily consolidates essential SEO and competitor research into one powerful platform. The customizable dashboards also let me create specialized reporting for clients.
5. LinkMiner
Finding guest posting and backlink opportunities is infinitely easier with LinkMiner. This Chrome extension highlights broken links across websites you visit, creating openings to suggest your own content as a replacement.
Contact site owners when you find their broken links, providing your article as a substitute. Not only can this lead to new backlinks, but it helps foster relationships with influencers in your space.
6. wpDataTables
wpDataTables is a WordPress plugin that allows you to easily create and manage tables and charts. The plugin offers a range of features, such as a drag-and-drop form builder, pre-built templates, and smart workflows to help you save time and effort.
With wpDataTables, you can create tables and charts in just a few minutes without writing any code. The plugin is 100% responsive and works on mobile, tablets, and desktops. wpDataTables also provides smart conditional logic to create high-performance tables and instant notifications to respond to leads quickly.
wpDataTables offers a range of plans to suit different needs and budgets. The plans are priced reasonably and offer a full range of features necessary for online table and chart creation. wpDataTables is the perfect tool for entrepreneurs and businesses looking to create smart tables and charts with ease.
7. TheHoth
The Hoth company provides different digital marketing services to help businesses grow and get more customers. Some of their main services include search engine optimization (SEO), pay-per-click advertising (PPC), and content writing.
In simpler terms, The Hoth team can help improve your website traffic, get more people clicking on your online ads, and create high-quality articles and blogs for your business. Specific services they provide are keyword research to optimize sites for Google searches, A/B testing for ads to improve conversions, link building to boost rankings, and managed accounts where they handle your online marketing for you. Their range of digital marketing offerings aims to get your website found more easily, drive more quality customers to your site, and ultimately boost your sales and revenue. The Hoth caters to organizations that don’t have the tools or experience to market themselves online effectively.
8. UberSuggest
UberSuggest is Neil Patel’s free keyword research tool, providing search volume estimates and related keywords for whatever seed keyword you input. Despite being fully free with unlimited queries, it churns out depressingly accurate data.
I tend to use UberSuggest in conjunction with my own tool to validate search volumes across both platforms. With its clean interface and clear, sortable keyword suggestions, UberSuggest remains a quick go-to even for basic research needs.
Marketing Tools
Shifting gears, let’s explore the latest marketing tools to amplify traffic, capture leads, and better understand your audience. These will prove invaluable as we seek new readers and revenue streams.
9. ConvertKit
ConvertKit specializes in email marketing and automation tailored for creators. More advanced than basic platforms like MailChimp, ConvertKit lets you create sequences, send broadcasts, and integrate with webinar and course platforms.
Metrics like open and click-through rates tell you what resonates with your audience. Split testing helps you continually refine your emails for more sign-ups and sales. And with its CRM and automation, you can segment contacts to target content that nurtures subscribers into customers.
Pricing starts at $29/month, making it quite affordable for established bloggers ready to scale their audience. I have sequences promoting my online courses that continue converting new students weekly.
10. Claude ai
Claude AI provides an easy way for websites to add a customized chatbot. A chatbot is an AI assistant that can answer visitor questions through conversational text chats. Website owners can use Claude to build their virtual helper without knowing computer coding.
Claude lets you create chatbots powered by artificial intelligence. You train your bot with questions and answers for your business or site topics. Claude’s bots can answer common queries, guide visitors around your site, support basic issues, or even complete actions like booking appointments. Everything happens right inside a live chat window that can pop up and assist visitors. The service aims to provide automated yet personalized assistance so real staff save time answering routine questions. Ultimately, Claude AI helps engage more customers and boost sales through 24/7 chatbot AI agents on your website.
11. Google Bard
Google Bard is a new experimental conversational AI service created by Google. It allows you to ask Bard questions on nearly any topic, and it will try to provide helpful answers and information.
Bard aims to be a helpful assistant you can chat with. For example, you can ask Bard questions like “How do plants grow?” or “What causes it to rain?” it will generate understandable explanations for you. Bard can summarize information, translate languages, suggest creative ideas, provide news updates, and more based purely on your questions. The system gets smarter the more people chat with it. While it has limitations currently, Google hopes Bard will someday be an easy way for people to learn or get information by simply asking questions and having a back-and-forth conversation with an AI helper.
12. Facebook Reels Insights
As TikTok-esque short-form video explodes across apps, Facebook added Reels last year to compete. One aspect many miss is the available Reels Insights about performance and audience.
The insights page shows which videos got the most reach, likes, and comments. It also reveals audience demographic data, including age, gender, location, and language. You can then use these learnings to fine-tune future reels based on what resonates best.
This free tool alone can exponentially improve content tailored to high-potential Facebook video niches and formats. As blogs expand beyond just text, these insights help tremendously in decoding ideal video strategies.
13. MightyShares
MightyShares makes share-worthy social media images for blog posts fully automatic. This WordPress plugin instantly creates beautiful, customized graphics to share each post, requiring nothing from you.
Shareability fuels visibility across social networks. By consistently featuring polished visuals for every article, MightyShares saves tons of tedious graphics creation work in Canva. Its set-and-forget automation also guarantees you never overlook sharing new stories on social media.
The free version adds watermarked images, while paid upgrades ($19+ per year) include more templates, custom fonts, and more. Give MightyShares’ free trial a test drive.
14. Buffer
Buffer remains one of my favorite social media tools for efficiently scheduling posts across networks like Facebook, Instagram, Twitter, LinkedIn, and more. Their Chrome extension also lets you rapidly add links to mid-browsing sessions to revisit later for sharing.
The most significant addition over the past year is Block Scheduling, which is game-changing for planning evergreen content queues around significant events. For example, you add recurrences like Cyber Monday offers, with posts evenly spacing themselves across that future period.
With its intuitive interface and generous free plan, Buffer removes all friction when planning and scheduling social promotion for blog content. Their browser extensions also caught up nicely to competitor tools.
15. Visme
Visme powers stunning data visualizations like infographics, presentations, and graphs with zero design expertise needed. The drag-and-drop editor and abundance of professionally made templates let you immediately create high-quality visual content.
Infographics especially perform tremendously well for capturing new audience share and links. With engaging stats and data points synthesized visually through images, Visme gives bloggers an easy avenue to amplify content through visual storytelling.
Their stock of photos, icons, and illustrations only adds to the professional polish possible for all skill levels. When trying to explain complex topics or surface key learnings, Visme simplifies translating concepts into compelling graphics.
16. Invideo
Invideo is a tool that helps you make videos more interesting and engaging by putting elements like images, graphics, stickers, and text animation on top of existing videos. For example, you could take a regular video and add some stickers, highlight certain parts with shapes and arrows, include pop-up text, and more to make it more visually appealing.
At its core, Invideo allows anyone to customize and enhance their videos without needing advanced video editing skills. You simply upload your initial video, then use their templates and drag-and-drop editor to add all sorts of illustrations, effects, captions, and text boxes on top. In just a few clicks, your original video becomes livelier and more captivating. So invideo essentially acts as an easy, automated way to level up your videos with dynamic visual elements and keep viewers hooked from start to finish. The only skill you really need is creativity!
17. Rytr
Rytr.me specializes in ultra-fast long-form writing by letting you choose content model types optimized for blogs, books, essays, stories, research papers, and more. It then asks you to define key elements of what you need to be written and takes over creating an initial draft.
During my test, Rytr produced a 2,200-word piece in about 3 minutes after briefly detailing the topic, target keywords, outline structure, and tone parameters. From there, you can insert custom text anywhere and make other tweaks prior to exporting the finished document.
If you want AI-powered content creation but find tools like Conversion.ai too unrestricted, Rytr’s guided approach may suit your needs better. Their $29/month starter plan won’t break the bank either.
18. Grammarly
After 13 years of blogging, I still use Grammarly nearly every day. Their writing assistant plugin catches misspellings, punctuation issues, passive voice, and other errors as you type away in Microsoft Office or Google Docs.
Grammarly explains each suggestion so you can improve your skills over time. Settings also allow adjusting detection sensitivity to eliminate false positives as needed. From students to executive bloggers, Grammarly satisfies writers of all kinds looking to level up their craft with the help of AI-powered feedback.
19. Hemingway Editor
The Hemingway online editor highlights complex, dense sentences and recommends simpler alternatives. Short, easy-to-read prose achieves greater comprehension, sharing, and rankings.
Paste any blog post or article in, and Hemingway will flag sentences ranked as “hard” or “very hard” to digest. It screens for dense phrases, overly intricate words, and rambling messaging. The built-in readability scoring also quantitatively shows you how understandable your content rates are.
For bloggers aiming to resonate with less technical audiences, writing with clarity and purpose should be non-negotiable. Hemingway Editor’s simplicity checks ensure your message comes across as intended.
20. Unsplash
Unsplash remains my go-to source for gorgeous, free stock photography. With over 1 million eye-catching images across every genre imaginable, it blows alternatives out of the water for legal, royalty-free blog imagery.
Studies continually show posts featuring visuals attract far greater engagement and sharing across channels like Pinterest. Unsplash makes consistently embedding photographic elements foolproof. And their powerful sorting, filtering, and search capabilities make hunting down on-brand graphics easier than ever.
For bloggers still manually creating every image with Canva, I beg you to start utilizing Unsplash’s seemingly infinite collection of photography. Finding the perfect pic takes seconds, saving tons of tedious graphics work.
21. Affilimate
Scaling affiliate sites juggling multiple networks quickly becomes convoluted, tracking income by channel, program, and even post/product. Affilimate solves this by centralizing reporting into one dashboard.
No matter the network size, Affilimate digests earnings across all partnerships. With robust analytics around trends, breakdowns, and segmentation, you gain visibility that is impossible otherwise. Attribution by product and page is also huge, instantly showing the highest ROI articles.
Affilimate’s simplified tracking and reporting will prove indispensable for bloggers managing affiliate links across channels. The 2-week free trial generously allows full-feature access to test out their platform.
22. Skimlinks
Skimlinks automates affiliate link conversion across your entire blog by partnering with 24,000+ merchant programs. Once approved, Skimlinks will swap common product links on your site for tracked affiliate versions without any work from you.
Their dashboard also reveals your highest earning posts, top converting products, Trending Products across the network, and broken links causing missed commissions. For bloggers overwhelmed by juggling so many partners independently, Skimlinks greatly condenses program tracking and insights.
23. Google Trends
Google Trends reveals top trending and highest traffic search queries in real-time. Monitoring hot topics here is invaluable for reacting quickly with related content while consumer interest is peaking.
The ability to compare multiple terms and see regional interest gives bloggers an always-updating pulse on rising trends. Keyword research tools show more historical patterns, but Trends spot emerging topics gaining momentum right now. Its website traffic tab also estimates search popularity, helping uncover new angles around key themes.
24. Canva
While around for years, Canva’s growth into a full-scale design platform earns its place here. Beyond creating standout graphics and social media images, Canva now powers animated videos, presentations, posters, logos, and more.
Their enormous template library spanning every visual format conceivable will inspire your creative ideas. With recent additions like photo editing, drawing tools, and sticking integrations, Canva’s push to dominate the content creation means bloggers benefit tremendously from the broad new creation capabilities.
25. QuillBot
QuillBot is a web tool that helps improve your writing. It uses artificial intelligence to rephrase and expand on your text. For example, if you paste a short paragraph into QuillBot, it will rewrite the text using synonyms and rearrange the sentences to say the same thing but in a fresh way.
Essentially, QuillBot is an AI writing assistant. It can rewrite and enhance any text you give it. You can use QuillBot to paraphrase an essay, summarize blocks of text, expand lists into complete sentences, change the tone or formality, and more. It helps you vary your word choice and prevents repetitive phrasing. Whether you’re a student struggling to finish an assignment, a professional crafting business content, or a blogger looking for inspiration, QuillBot provides an easy way to edit and build upon your writing. It aims to help anyone become a stronger and more creative writer through the power of its advanced language technology.
26. Hubspot Blog Topic Generator
HubSpot is an all-in-one software platform that helps businesses with marketing, sales, and customer service operations. Instead of having different tools for things like email, social media, contacts, and tracking results, HubSpot combines it all into a single dashboard.
Some examples of what HubSpot can do are automate and schedule emails and social media posts, store a unified contacts database, host websites and blogs, take payments and book appointments, provide live chat support, track leads and sales opportunities, run ads, and more. All data feeds into powerful analytics dashboards. HubSpot aims to have every tool a growing company needs to run day-to-day tasks, analyze performance, and optimize its business growth in key areas like search traffic, lead generation, and sales. The all-in-one integrated platform replacing individual tools is explicitly tailored to scaling companies.
In summary, HubSpot is a centralized marketing, sales, and service platform with integrated tools, data, and insights that modern businesses need as they expand their operations. I aimed to focus on the consolidation and analytical benefits they offer companies wanting to manage and improve customer acquisition and retention. Please let me know if you need any clarification or have additional questions!
27. Portent Idea Factory
Portent is an online company that provides different digital marketing tools and services to help businesses promote themselves online and attract more website visitors.
Some specific services Portent offers include search engine optimization (SEO) to improve Google rankings, content writing to create blogs and articles, and pay-per-click (PPC) management to set up and optimize ads. Portent also provides website audits to recommend improvements, analytics dashboards to track performance, technical website fixes to boost speed and security, and general digital strategy consulting. Essentially, Portent has diverse professional services and software aimed at increasing a business’s leads, traffic, and customers through enhanced online visibility and optimization. They market themselves as an expert extension of a company’s digital marketing and web teams.
In summary, Portent provides digital services and tools to elevate a business’s online presence to acquire more quality customers. This covers technical enhancements, content creation, strategic consulting, and advertising management, catering to customer acquisition needs. Please let me know if you need any clarification or have additional questions!
28. INK Content Optimization
Ink For All is an online printing service that makes creating and ordering professionally printed materials easy for anyone. Rather than needing design experience or ordering through traditional printers, Ink For All streamlines the process using their website and apps.
Some examples of products you can customize and print through Ink for All include business cards, postcards, brochures, stickers, flyers, and banners. All you need to do is select a starting template or upload your design, customize it by dragging in images and entering text, place the order, and wait for delivery. Ink For All handles professional printing, paper choices, quality checks, and shipping entirely online. The service aims to provide an intuitive way for people and business owners to bring their visual ideas to life with physical print marketing materials without headaches or special software knowledge.
In summary, Ink For All offers easy online graphic design tools combined with professional printing and shipping for all print collateral, from business cards to banners. Online automation helps everyday people and business marketers create and order stunning printed graphics straight from the website. Please let me know if you need any clarification or have additional questions!
29. WordTune
Wordtune is a writing web app that helps you compose better content by giving you suggestions as you type. Its technology acts like an auto-complete for sentences, assisting average writers to create text that reads professionally.
As you write in Wordtune, it dynamically generates alternative ways to phrase each sentence to sound more straightforward and more eloquent. You can cycle through the options it provides mid-sentence and choose the one that best conveys what you want to say. Features like text expansion and vocabulary synonyms further enhance your writing. Wordtune essentially tries to mimic the revisions an editor might provide as you go. Constantly generating optimized suggestions tailored to the context can help any writer turn rough drafts into polished prose. The service aims to boost writing quality and save editing time through its unique AI recommendation system.
In summary, Wordtune utilizes AI to provide real-time writing recommendations so writers can easily craft eloquent, expressive text. It is an interactive editor that enhances your writing style and clarity as you type.
30. Shortly Readable
Shortly, AI is software that uses artificial intelligence to summarize long pieces of text for you in just a few seconds. It’s useful for anyone who needs to quickly understand key information from research papers, articles, documents, and more without reading whole pages.
You simply copy and paste any lengthy content into their website’s Shortly AI summarizer tool. It will scan and analyze the text, then create a shortened version, keeping only the most important sentences. The summary length can be customized to your needs. Their algorithm figures out the central topic and main points automatically. So rather than spending lots of time reading lengthy documents, Shortly AI lets you get a concise summary you can absorb in minutes. It makes reading and research tasks much more efficient by letting AI pull out key details. The service aims to save people time and effort while ensuring they fully grasp key information.
Shortly, AI provides an automated summarization tool that reduces long text to concise overviews, using AI to determine relevance and brevity. It helps absorb information much quicker.
31. DALL-E 2 and DALL-E 3
DALL-E is a new artificial intelligence system that can create unique images and digital art from a text description. Instead of needing an artist to draw or design graphics, DALL-E lets anyone instantly generate images by typing a phrase.
For example, you can ask DALL-E to “create a photo of a baby koala drinking a milkshake in space.” It will generate a completely original, photorealistic image visualizing that idea in seconds. DALL-E can mix random concepts, design products, create logos, modify photos, and more based purely on the text you provide. Under the hood, it has been trained on millions of images and their captions to learn these connections. While it sometimes creates strange interpretations, DALL-E creates imagination and creativity for people without graphic design experience. The system demonstrates a futuristic AI capability to turn text into extremely realistic images through its language and image training process.
In summary, DALL-E leverages AI to generate highly advanced digital images and art by automatically interpreting text descriptions. It opens new creative possibilities for people without artistic/technical skills.
32. Midjourney
Midjourney is an artificial intelligence system that creates original images and 3D models simply from text prompts. Instead of needing graphic design experience, Midjourney lets anyone generate custom digital art and imagery by describing what they want to see.
For example, you can prompt Midjourney to “make a cute baby panda playing guitar in a field of flowers.” In seconds, it will synthesize a completely new surreal image visualizing that idea. Midjourney brings images and concepts to life. It can design logos, rooms, book covers, fashion looks, and more based on descriptive input text. Under the hood, Midjourney has been trained on millions of photos, illustrations, and 3D models to understand and render requested concepts. While results vary, the tool allows creativity and imagination for those without artistic skills. You can continually refine and guide each image towards your vision. Essentially, Midjourney aims to make digital art and design generation accessible to all by harnessing the potential of AI.
In summary, Midjourney leverages AI to generate custom digital artwork, images, and 3D models simply from text prompt descriptions. It expands creative possibilities to non-artists.
33. Descript
Descript is an audio editing tool that allows anyone to edit podcasts, interviews, videos, and other text recordings. Rather than needing to learn complex audio editing software, Descript makes it as easy as typing and deleting words.
For example, you can upload an audio or video file into Descript, and it will automatically transcribe everything said into an editable text document. To make edits, you revise the text transcription like a regular document. Delete filler words, fix mistakes, insert new sentences – whatever you change in the text will be reflected in the audio. Descript handles splicing, editing, and adjustments behind the scenes using the text. So, no more struggling with waveforms – Descript aims to provide intuitive, text-based audio and video editing to professionals and amateurs alike. This new approach harnesses transcription and speech synthesis to simplify podcast, video, and all audio production needs.
Descript offers text-based audio/video editing powered by its integrated transcription and audio reconstruction capabilities. Edits on the text script automatically adjust the final recording.
34. All in one SEO
AIOSEO is a powerful and user-friendly WordPress SEO plugin that helps you improve your website’s search rankings. With over 3 million users, AIOSEO is the world’s best all-in-one SEO plugin for WordPress. It offers a range of features that make optimizing your website for search engines easy, including TruSEO On-Page Analysis, Rich Snippets Schema, Smart Sitemaps, Local SEO, WooCommerce SEO, and Advanced SEO Modules.
AIOSEO’s TruSEO On-Page Analysis feature lets you easily add title tags, meta descriptions, keywords, and everything else you need for proper on-page SEO optimization. The Rich Snippets Schema feature offers complete support for schema markup so you can get more clicks and traffic with rich snippets. The Smart Sitemaps feature automatically generates a WordPress XML sitemap and notifies all search engines of updates. The Local SEO feature gives you all the tools to improve your local SEO and rank higher on Google Maps. The WooCommerce SEO feature provides advanced eCommerce SEO support for WooCommerce to optimize product pages, product categories, and more.
AIOSEO also includes a video sitemap generator, so you can rank in Google’s video carousel widget and grow your traffic. You can easily control how your content and thumbnails look on Facebook, Twitter, and other social media networks with AIOSEO’s Social Media Integration feature. The Link Assistant feature provides relevant suggestions for adding internal links to older content and finding any orphaned posts with no internal links. The SEO Audit Checklist feature analyzes your entire WordPress site to detect critical errors and provides actionable insights to boost your SEO and get more traffic.
Finally, AIOSEO’s Redirection Manager feature allows you to easily create and manage redirects for broken links to avoid confusing search engines or losing valuable backlinks. With AIOSEO, you can get comprehensive reports on content decay, monitor your website’s performance, keep track of keyword rankings, and analyze search trends. Stay one step ahead of the competition and optimize your website’s SEO strategy for maximum results with AIOSEO.
35. MarketMuse
MarketMuse is an AI-powered writing assistant that helps you create high-quality website content optimized for search engines like Google. Rather than struggling to research topics and incorporate the right keyphrases manually, MarketMuse handles the hard work for you.
Writing blogs, articles, or product descriptions in MarketMuse will automatically generate relevant keywords and sentences as suggestions that you can accept or customize as you type. This ensures all content includes the phrases and terminology that internet searchers are actually looking for. MarketMuse pulls search data like monthly lookups to guide its recommendations.
Additionally, it will rewrite long blocks of text to be more succinct using AI summarization capabilities. The service aims to eliminate the guesswork from effective content creation – continually supplying you with research-backed information and phrasing. With MarketMuse handling the optimization, you only worry about writing engaging material, not SEO specifics.
MarketMuse is an AI writing tool focused specifically on creating website content tailored for high search engine rankings through its automated keyphrase recommendations and readability improvements.
36. Contentyze
Contentyze is an AI tool that improves and expands on written text to make it more engaging and optimized for search engines. It helps create website content that drives more visitors and traffic.
Contentyze has automated tools that can take a draft blog post or article outline and rewrite sentences to be more precise and of higher quality. It provides intelligent suggestions to add related keywords and links naturally throughout. The AI will expand on critical points, pulling facts and data from trusted online sources. Additionally, Contentyze will run readability assessments and offer further refinements.
All the recommendations aim to shape the content for better reader engagement, SEO awareness, and conversions. Essentially, the platform streamlines crafting blogs, websites, and ad copy all in one intuitive editor instead of switching between various tools. It combines the essential elements to resonate with audiences and improve online visibility.
Contentyze provides an integrated AI content editor that enhances text for user engagement and search engine friendliness through its automated analysis and revision capabilities tailored to digital marketing needs.
37. Pitchbox
Pitchbox is an online tool that helps you create stunning slideshow presentations using artificial intelligence. Instead of needing design experience, Pitchbox allows anyone to make professional decks easily.
To create a presentation in Pitchbox, you describe in standard sentences what you want each slide to show. For example, you could say, “First slide – show a pie chart of our sales numbers.” Pitchbox automatically generates a visual slide with your perfectly formatted pie chart data. You can create a complete branded deck by describing each slide’s content in plain language.
Pitchbox has hundreds of templates, from business overviews to product pitches to creative portfolios. It uses AI to handle graphic design, image searching, infographic & logo creation, color schemes, layouts, and more based on your descriptions. Essentially, Pitchbox aims to handle all the design work so you can focus efforts more on presentation strategy and writing using its AI-based creation technology.
In summary, Pitchbox leverages AI generation to design full presentation decks based on plain text slide descriptions. It makes professional pitch and branded content deck creation easy for general productivity.
38. Screaming Frog SEO Spider Tool
Screaming Frog SEO Spider is a website crawling tool for Search Engine Optimization (SEO). Essentially, the software quickly crawls through an entire website, checking user-facing pages and behind-the-scenes technical elements.
The Screaming Frog crawler analyzes broken links, page load speeds, accessibility issues, meta titles/descriptions, on-page content around keywords, internal search setup, canonical tags, and much more. All this information gets pulled into detailed reports showing opportunities in areas like site indexing, duplicate content, site migrations, tackling penalties, optimizing pages for topics with high search volume, and more.
Screaming Frog shines a light on the health of websites and discovers fixes to increase organic search traffic by surfacing technical problems or improvements site owners may not have seen crawling manually alone. The software aims to provide insight and solutions into flaws hindering discoverability and search visibility.
In summary, Screaming Frog is a crawler that gives digital marketers and webmasters a comprehensive technical SEO audit of websites to diagnose issues hurting organic rankings and provide specific fixes to optimize full discoverability.
39. Bing AI Chat and Bing Create & Image Generator
Bing Chat is a feature of the Bing search engine that allows users to converse with an AI chatbot instead of typing in search queries. It provides intelligent search results, interactive searching, visual exploration, and personalized suggestions. Bing Chat is accessible through Microsoft Edge, and it can be accessed by signing into Microsoft Edge and selecting the Bing Chat icon in the browser toolbar. Feature availability and functionality may vary by device type, market, and browser version.
Bing Chat has all the search and creative capabilities of Bing Chat and more. In the sidebar, Chat can also perform searches and answers about the page you’re viewing. For example, it can help you find what wine to pair with a recipe, compare two products, or summarize a report. Chat can also compare products in your sidebar and create a table to help you compare. While Chat can help you compare products, Shopping in the sidebar can help you get the best deal. Bing Chat in the Microsoft Edge sidebar will soon be able to summarize more types of documents, including PDFs, Word documents, and longer website content, making it easier to consume dense content online.
Bing Chat is a powerful tool that can help you find exactly what you’re looking for while on the go. You can access Bing Chat from the Microsoft Edge app menu, making it easier to take your chat with you, ask questions about web content, and find exactly what you’re looking for while on the go. Bing Chat is also available on the Microsoft Edge mobile app, where you can ask Bing Chat questions and summarize and review content when you view a PDF in your Edge mobile browser.
And
Bing Create is a feature of the Bing search engine that allows users to create and share their custom search results. It provides a platform for users to curate and share their search results, including web pages, images, videos, and more. Bing Create is accessible through Microsoft Edge, and it can be accessed by signing into Microsoft Edge and selecting the Bing Create icon in the browser toolbar. Feature availability and functionality may vary by device type, market, and browser version.
Bing Create has all the search and creative capabilities of Bing and more. It allows users to create their custom search results by adding web pages, images, videos, and more to their search results. Users can also share their custom search results with others, making it easy to collaborate and share information. Bing Create is a powerful tool that can help you find exactly what you want while on the go. You can access Bing Create from the Microsoft Edge app menu, making it easier to take your custom search results, ask questions about web content, and find exactly what you’re looking for while on the go.
Bing Create is an excellent tool for anyone who wants to curate and share their search results. Whether you’re a student, researcher, or just someone who wants to share information with others, Bing Create makes it easy to create and share your own custom search results. With Bing Create, you can create your custom search results in just a few clicks, making it easy to find precisely what you’re looking for
40. Linkody
Linkody combines AI and human intelligence to research link-building tactics and trends.
Linkody is a search engine optimization (SEO) tool that allows users to monitor and track their backlinks. It will enable users to track their backlinks, monitor their competitors’ backlinks, and get insights into their link-building strategies. Linkody is accessible through its website and can be accessed by signing up for an account on the website. Feature availability and functionality may vary by device type, market, and browser version.
Linkody has all a search engine’s search and creative capabilities and more. It allows users to track their backlinks and monitor their competitors’ backlinks. Users can also get insights into their link-building strategies, making it easy to improve their SEO. Linkody is a powerful tool that can help you find exactly what you’re looking for while on the go. You can access Linkody from the website, making it easier to take your backlink tracking, ask questions about web content, and find exactly what you’re looking for while on the go.
Linkody is an excellent tool for anyone who wants to improve their SEO. Whether you’re a blogger, marketer, or just someone who wants to improve their website’s search engine ranking, Linkody makes it easy to track your backlinks and monitor your competitors’ backlinks. This fuels data-driven link strategy advisory quantifying opportunity sizing, topically relevant sites, and outreach prioritization.
41. Jaaxy
Jaaxy is an online keyword research tool used to find and analyze words and phrases people search for in search engines like Google. Finding the best keywords is essential for writing quality blog content or search ads.
Jaaxy provides keyword ideas related to your business and refines them further based on search volume, competition levels, and related searches. You can get insights tailored for SEO content, pay-per-click ads, and product marketing keywords. Analyze search trends over time, highlight buyer keywords, batch track ranking positions, and more. Jaaxy essentially simplifies and consolidates finding high-potential, high-value search queries to create content around. It empowers businesses to understand user intent through keywords to optimize websites, ads, and messaging. Rather than relying on guesswork, Jaaxy gives data-driven keyword guidance to drive discovery and traffic.
In summary, Jaaxy is an intelligent keyword research and analysis platform providing actionable data to inform better website discoverability, content, and marketing campaigns.
42. NichePursuits
NichePursuits expedites discovering profitable affiliate niches and corresponding blogs ideal for partnership and integration, serving shared audiences interested in monetized recommendations.
I hope this gives you some new cutting-edge AI tools to test that can significantly move the needle with your overall organic growth through better SEO, strategic guest posting, and mutually beneficial affiliate partnerships.
43. LinkedIn Newsletters
LinkedIn added creator newsletters last year, allowing anyone to self-publish customized content to their professional network. Complete custom branding, analytics, lead generation, and community-building features make them robust publishing hubs compared to Facebook’s analog.
Nearly 3 million people across industries reportedly subscribe to LinkedIn newsletters already. Tapping into these engaged, business-minded readers presents a significant opportunity for creator-centric bloggers. I’ll soon launch my newsletter to complement blog content and foster deeper reader loyalty.
44. Tailwind
Simplifying Pinterest engagement at scale, Tailwind is the highest-rated Pinterest automation tool that self-executes captivating posts. After connecting Pinterest Analytics, Tailwind studies your best content and then uses that intel to pin it accordingly.
You can also create smart schedules targeting key traffic windows. Board organization keeps your profile looking polished, too. For bloggers still manually pinning, Tailwind introduces completely hands-off Pinterest automation so you focus energy only on creation.
45. CopyMatic
Copymatic is an AI-powered writing tool that can help you generate high-quality content quickly and easily. It uses the GPT-3/4 AI language model to create unique and engaging copy for various purposes, including blog posts, website copy, social media ads, etc. With over 80 templates, Copymatic.ai Can help you write anything from long-form articles to product descriptions in seconds.
Copymatic is accessible through its website and can be accessed by signing up for an account on the website. Once you’re signed up, you can use the tool immediately. Select a writing tool from the vast array of AI tools available, fill in your product details, and let the AI generate unique and human-like content in seconds. You can also adjust the creativity level or the tone of voice to generate the perfect copy for your business.
Copymatic is a powerful tool that can help you save time and effort while creating high-quality content. Whether you’re a blogger, marketer, or someone who wants to improve your writing skills, Copymatic makes it easy to generate unique and engaging content quickly and easily.
46. LinkinBio
LinkinBio solved the long-standing Instagram link limitation in user bios by creating a customizable landing page linking to your important content. Populate sections with lead magnets, products, and external profiles you want to direct traffic toward from IG.
LinkinBio’s slick personal domain and menu creation removes friction for driving site visitors from Instagram. For bloggers focused on monetization, this tool lets you prominently showcase income offerings like online courses or membership options from your bio.
47. Creator Suite
Facebook Creator Suite aims to simplify managing Pages and Profiles across FB and IG and digesting social performance. Cleaner interfaces add usability to cumbersome business tools in native apps.
Content planning and publishing routes content seamlessly to both networks in one flow. Unified in Boxing centralizes comments, mentions, and messages, too. And the tool compiles analytics and insights to see how content resonates on each platform.
This free product demonstrates Facebook’s commitment to serving digital creators and bloggers focused on engaging audiences using their apps. The streamlining around multi-channel posting and messaging alone makes it a valuable addition.
48. Linkilo
Linkilo is a specialized platform focused on optimizing internal linking strategies for websites. This website offers tools and services that are particularly beneficial for enhancing SEO (Search Engine Optimization) through intelligent link management. Here’s a breakdown of what Linkilo provides:
- Efficient Internal Link Management: The primary service offered by Linkilo is the management of internal links within a website. Internal links are hyperlinks that connect one page of a website to another page within the same website. These links are crucial for SEO as they help structure a website and improve its navigability, positively impacting search engine rankings. Linkilo provides an efficient system to manage these links, ensuring they are organized and effectively used to enhance a site’s SEO.
- SEO Improvement through Intelligent Linking: Linkilo emphasizes using intelligence in linking strategies. This means that the platform helps create and manage internal links and ensures that these links are strategically placed and used. By analyzing the content and structure of a website, Linkilo suggests the most effective ways to implement internal links, thereby improving the site’s overall SEO performance.
- User-Friendly Interface and Tools: The platform is designed to be user-friendly, catering to SEO professionals and those new to SEO. It simplifies the often complex process of internal link management, making it accessible for users with varying levels of SEO expertise. This approach allows website owners and content creators to take control of their internal linking strategy without needing deep technical knowledge.
In summary, Linkilo is a platform that specializes in optimizing internal linking for websites. It offers tools and services for efficient link management and uses intelligent strategies to improve a website’s SEO. Its user-friendly interface makes it accessible to a wide range of users, from SEO experts to beginners.
49. Metricool
Metricool fills the gap left by Google Search Console, which only reports keyword ranking progress monthly. Their rank tracker refreshes daily, delivering zero lag visibility into positioning shifts across search engines.
Daily changes allow rapidly testing new optimization tweaks before losing months of potential traffic waiting on Search Console data. Competitor tracking also uncovers new pyramid semantic opportunities clustering around your targets. For impatience bloggers like me, Metricool is my ranking report accelerator.
50. HeyGen
HeyGen is an innovative video platform that harnesses the power of generative AI to streamline your video creation process. The platform offers a range of features that allow you to create professional videos without the need for expensive equipment, inconsistent actors, complex editing tools, or third-party teams.
With HeyGen, you can turn texts into professional videos with AI-generated avatars and voices. The platform offers over 100 AI avatars covering different ethnicities, ages, poses, and clothes and over 300 high-quality voices in 40+ languages. You can also create your avatar or use a dynamically animated photograph.
HeyGen is ideal for product marketing, content marketing, sales outreach, and learning and development. You can produce engaging and concise product videos and craft captivating product releases, updates, and explainer videos that captivate your audience from start to finish. You can also supercharge your content calendar with videos and craft compelling content through videos, articles, and social media to communicate your brand’s value, connect with your audience, and drive traffic.
HeyGen also allows you to create personalized outreach videos at scale and elevate your outreach game with compelling and tailored video content that sets your SDRs up for success in their efforts to drive growth and revenue. Finally, you can create training videos your team will watch, professional videos without mics, cameras, or actors, and use AI avatars for high-quality content in 40+ languages.
HeyGen is SOC 2 compliant, an industry standard for data protection, and built with your privacy and protection in mind. You can trust HeyGen with your valuable data. HeyGen also has an ethical approach to AI, emphasizing acceptable use and transparency.
51. Uberflip
Convert Experiences from Uberflip create personalized lead capture and conversion microsites with no dev resources required. Build everything from quizzes to assessments, guides, calculators, and more using their library of beautifully pre-made templates.
Pages integrate directly with marketing platforms like HubSpot, Salesforce, and Marketo with just a few clicks. Uberflip then tracks engagement across devices to model visitor experiences, optimizing conversion performance over time. No coding. No third-party apps. Just results.
52. Drip
Drip advances marketing automation dramatically from essential email platforms like Mailchimp. Workflows react to reader behaviors in real time with conditional rules, personalization, and scheduling tailored to each subscriber.
Custom conversion funnels nurture leads across multiple stages, whether they read your latest email, click a link, or purchase through your site. Integrations with e-commerce platforms also let you reward customer milestones and activity with special incentives via Drip. No more stagnant batch-and-blast emails.
53. Quora Spaces
Quora presented an opportunity for creators to get discovered by and engage audiences around niche topics. Spaces are mini-interest-based communities allowing for intimate, interactive Q&A between you and readers.
Optimizing a Space for maximum appeal gives bloggers a new channel for showcasing expertise and owning categories tied closely to their content focus. The built-in readership hungering for insights around your themes offers a receptive platform for converting engaged followers.
54. ContentStudio
ContentStudio is an AI writing assistant that helps you create high-quality blog posts and other content faster. It provides research, outlines, drafts, headlines, and more with just a few clicks.
The key features include article writing, rewriting and summarization, SEO optimization, plagiarism checking, and image searching. It’s designed specifically for bloggers and marketers to save time while producing great content.
55. Clean Email
CleanEmail is an online service designed to help users manage and organize their email inboxes. It offers a range of features to make email management more efficient and less overwhelming. Here’s a detailed breakdown of what CleanEmail.com provides:
- Email Cleaning and Organization: The primary function of Clean Email is to assist users in cleaning and organizing their email inboxes. This service benefits those who receive many emails and find it challenging to keep their inbox organized. Clean Email offers tools to quickly sort, review, and delete thousands of emails simultaneously, helping users declutter their inboxes effectively. It also allows users to unsubscribe from unwanted email subscriptions, reducing inbox clutter efficiently.
- Advanced Features for Email Management: Clean Email has advanced features beyond basic inbox cleaning. Users can create custom rules and filters to automate the organization of incoming emails. This means that emails can be automatically grouped, labeled, or archived based on user-defined criteria, making managing large volumes of emails easier. The service also provides options to manage multiple email accounts from a single interface, enhancing convenience for users with more than one email account.
- User-Friendly and Efficient: The platform is designed to be user-friendly, catering to individuals and professionals. It emphasizes efficiency, allowing users to regain control of their inboxes with minimal effort. The interface is intuitive, making it easy for anyone, regardless of technical expertise, to navigate and use the service effectively.
In summary, CleanEmail offers a comprehensive solution for email management and organization. Its features are designed to help users efficiently clean and organize their inboxes, manage multiple email accounts, and automate email sorting. The platform is user-friendly and suitable for both personal and professional use, addressing the common challenge of email overload.
56. Substack
Substack empowers bloggers to publish newsletters and manage paid subscriptions easily. You can import email subscribers, customize branding and layouts, and accept membership payments.
Robust analytics provide subscriber engagement data to optimize newsletter content. Integration with Mailchimp also allows seamlessly migrating existing email lists over to Substack. It’s completely free to get started.
57. Meta Business Suite
The Meta Business Suite consolidates key tools for managing social media presence across Facebook, Instagram, and Messenger. Core functions for publishing, engagement, ads, commerce, and analytics reduce time spent toggling between business products.
The unified inbox also enables streamlining community response efforts in one place. As bloggers scale pages and followers across an expanding Metaverse presence, this free consolidation hub delivers much-needed simplification.
58. Synthesia
Synthesia is an AI-powered video creation platform that enables users to generate high-quality videos with ease. The platform offers a range of features that allow you to create professional videos without the need for expensive equipment, inconsistent actors, complex editing tools, or third-party teams. With Synthesia, you can turn your text into videos in minutes with natural-sounding AI voices and diverse AI avatars that mimic human-like expressions and movements. The platform offers over 140 AI avatars covering different ethnicities, ages, poses, and clothes and over 120 high-quality voices available in 40+ languages. You can also create your own avatar or use a dynamically animated photograph.
Synthesia is ideal for teams who create training videos at scale, learning and development, sales enablement, customer service, information security, and marketing. You can replace boring text, PowerPoints, and PDFs with engaging videos your team will love. Synthesia allows you to produce engaging and concise product videos craft captivating product releases, updates, and explainer videos that captivate your audience from start to finish. You can supercharge your content calendar with videos and craft compelling content through videos, articles, and social media to communicate your brand’s value, connect with your audience, and drive traffic. Synthesia also allows you to create personalized outreach videos at scale and elevate your outreach game with compelling and tailored video content that sets your SDRs up for success in their efforts to drive growth and revenue. Finally, you can create training videos your team will actually watch, create professional videos without mics, cameras, or actors, and use AI avatars for high-quality content in 40+ languages.
Synthesia is SOC 2 compliant, an industry standard for data protection, and built with your privacy and protection in mind. You can trust Synthesia with your valuable data. Synthesia also has an ethical approach to AI, emphasizing acceptable use and transparency.
59. Fliki
Fliki is a video creation platform that uses AI to help you create stunning videos in minutes. The platform offers a range of features that allow you to create professional videos without the need for expensive equipment, inconsistent actors, complex editing tools, or third-party teams. With Fliki, you can turn your text into videos with natural-sounding AI voices and diverse AI avatars that mimic human-like expressions and movements. The platform offers over 1300 ultra-realistic voices covering different languages and dialects, ensuring that you can create videos with voiceovers in your desired language with ease. You can also create your own avatar or use a dynamically animated photograph.
Fliki is ideal for creating educational videos, explainers, product demos, social media content, YouTube videos, TikTok Reels, and video ads. You can personalize your AI voice with a choice from a diverse range of more than 2,000 human-sounding voices in over 75 languages. Fliki’s text-to-video tool is fully web-based, so you only need a device with internet access and a browser to create, edit, and publish your videos. You can export your videos in formats like MP4. Fliki also offers a free tier that allows users to explore text-to-voice and text-to-video features without any cost. You can generate 5 minutes of free audio and video content per month. However, certain advanced features and premium AI capabilities may require a paid subscription.
Fliki stands out from other tools because it combines text-to-video AI and text-to-speech AI capabilities to give you an all-in-one platform for your content creation needs. Fliki helps you create visually captivating videos with professional-grade voiceovers, all in one place. In addition, Fliki takes pride in its exceptional AI Voices and Voice Clones, which are known for their superior quality. Fliki is SOC 2 compliant, an industry standard for data protection, and built with your privacy and protection in mind. You can trust Fliki with your valuable data.
60. PayPal Commerce Platform
Similar to Shopify, PayPal Commerce Platform makes launching customized online stores fast without needing advanced technical skills. But it goes further allowing bloggers to also directly accept payments, invoice clients, manage subscriptions, and more.
Flexible monetization and seamless global payment processing uniquely position this to become a scalable hub for consolidating all blogger business operations in one cloud-based ecosystem. Now through 2023, PayPal is waiving all platform fees, making adoption nearly indispensable.
61. CircleBoom
Circleboom is a comprehensive social media management tool designed to streamline and enhance the way individuals, brands, and small to medium-sized businesses (SMBs) manage their social media presence. Here’s a detailed breakdown of what Circleboom offers:
- Social Media Post Management: The core service of Circleboom is to provide a unified platform for designing, planning, automating, and posting or scheduling social media content. This feature is incredibly useful for users who manage multiple social media accounts or need to maintain a consistent posting schedule across different platforms. The tool allows for the creation and scheduling of posts in advance, which helps in maintaining a steady and strategic presence on social media. This is particularly beneficial for brands and SMBs that aim to engage their audience regularly without the need to be online all the time.
- Advanced Social Media Features: Beyond basic post-scheduling, Circleboom offers a range of advanced features to optimize social media management. This includes follower and unfollower tracking, which is essential for understanding audience engagement and growth. The platform also provides detailed Twitter analytics, hashtag tracking, and tools to find inactive accounts. For Twitter users, Circleboom offers functionalities like the ability to delete tweets and manage followers more effectively. These features are designed to give users a more in-depth understanding of their social media performance and audience behavior.
- User-Friendly Interface and Accessibility: Circleboom emphasizes a user-friendly experience, making it accessible for users regardless of their technical expertise. The interface is intuitive, allowing for easy navigation and use of its features. This approach makes it suitable for a wide range of users, from individuals looking to manage their personal social media accounts more effectively to brands and SMBs seeking to strengthen their social network and online presence.
In summary, Circleboom is a versatile social media management tool that offers post-scheduling, advanced analytics, and audience engagement features. It is designed to help users, brands, and SMBs grow and strengthen their social network by providing an efficient and user-friendly platform for managing various aspects of social media.
62. Media.net
Media.net operates a huge contextual advertising network that bloggers can join to monetize articles through automatically matched, high-quality display ads. Because ads closely relate to the page content itself, they complement the reader experience instead of detracting.
Faster payments, strong advertiser partnerships, and the ability to serve non-invasive commercial messages make Media.net an industry leader that delivers premium results for publishers seeking to amplify earnings. Their optimization tools also empower tailoring strategies, maximizing both relevance and RPM.
63. Payoneer
Getting paid quickly and securely from advertisers, affiliate programs, and other monetization partners persists as a headache for bloggers globally. Payoneer aims to solve this through virtual global bank accounts that receive funds in any currency, stored as USD/EUR, or easily withdrawn locally.
Serving over 4 million freelancers, online sellers, and publishers in 200 regions, Payoneer’s financial services provide peace of mind that earnings flow reliably no matter where bloggers or partners reside. I personally faced enough delayed payments over 13 years of blogging, making Payoneer’s promise of faster payout critical insurance.
64. Teachable Presell Page Builder
Teachable powers bloggers are creating and selling online courses, but their newer Presell Page Builder stands out by making high-converting sales pages drag-and-drop easy. Dynamically personalize messaging to boost enrollment rates and revenue.
Copy blocks, multimedia embedding, custom colors, and other branding elements take just minutes to mix and match before publishing beautiful promotion pages natively hosted on your online school’s domain. As bloggers expand their expertise into premium digital learning products, elevating marketing capabilities grows increasingly crucial for viability against mainstream competition.
65. MightyNetworks
Between email lists, social media, and blog readers, bloggers accumulate sizable yet disjointed audiences spread across disconnected channels. MightyNetworks emerges as the tool to unite these groups under one virtual roof, bolstering community engagement.
Mighty Networks is a platform that helps you create and grow your own online community, courses, and memberships. You can use Mighty Networks to share your knowledge, passion, and expertise with your audience and monetize your content with paid subscriptions or products. Mighty Networks offers a range of features that allow you to customize your community, design engaging courses, host live events, Livestream videos, and more. You can also upgrade your community to your own branded mobile apps and website, giving you more control and visibility over your brand.
Mighty Networks is ideal for creators, entrepreneurs, coaches, teachers, authors, podcasters, and anyone who wants to build a loyal fan base and generate income from their online presence. You can join Mighty Networks for free and start creating your own community in minutes.
Centralized membership perks, access controls, live streaming, chat forums, and dynamic user roles are just some capabilities activating bloggers’ dormant followings into unified brand supporters with true belonging and value. The codes and themes empower extensive customization, too, for unique models per creator.
66. Quuu Promote
Quuu Promote distributes blog content to an established network of publishers willing to re-share quality articles with their own audiences in exchange for earnings and attribution.
This amplification exchange helps bloggers syndicate material, driving greater readership and backlinks through credible third parties. Curating lists of your best articles allows Quuu’s partners to cherry-pick the exact pieces for respective niche followers. The associated credit system creates mutual value.
67. Slideshare
Slideshare allows uploading PowerPoint presentations, infographics, and other captivating visual content to embed or share anywhere. Natively, support across leading platforms keeps material broadly discoverable.
Upload once; syndicate everywhere remains the win with Slideshare. Their popularity amongst searchers earns high domain authority rankings, passing linkage benefits to featured domain names and social profiles. Bloggers investing heavily in data visualizations or speaking decks gain tremendous leverage, repurposing those assets with Slideshare integration.
68. HexoWatch
Hexowatch is an AI-powered tool designed to monitor and archive changes on websites. It serves as a versatile assistant for anyone needing to track updates or alterations on various web pages. Here’s a detailed breakdown of what Hexowatch offers:
- Comprehensive Website Monitoring: The primary service of Hexowatch is to monitor websites for any changes. This includes a wide range of aspects such as visual changes, content updates, price modifications, source code alterations, technology shifts, and even WHOIS record changes. This feature is particularly useful for businesses and individuals who need to keep an eye on competitors’ websites, track price changes, monitor content updates, or stay informed about any significant changes to websites of interest. The AI-driven nature of Hexowatch allows for accurate and timely detection of changes, ensuring users are promptly notified.
- Versatile Monitoring Options and Archiving: Hexowatch provides various monitoring options tailored to different needs. Users can choose to monitor visual elements, which is helpful for seeing layout changes, or focus on content and HTML for textual and structural modifications. Additionally, the tool offers technology monitoring, which alerts users to changes in the backend technologies of a site, and availability monitoring to check if a website is up and running. Beyond monitoring, Hexowatch also archives the changes, allowing users to review historical data and understand the evolution of a website over time.
- User-Friendly Interface and Accessibility: The platform is designed to be user-friendly, catering to both technical and non-technical users. Its interface is straightforward, making it easy for anyone to set up and start monitoring websites without needing advanced technical knowledge. This accessibility makes Hexowatch a valuable tool for a wide range of users, from business owners and marketers to researchers and web enthusiasts.
In summary, Hexowatch offers a comprehensive solution for monitoring and archiving website changes. Its AI-powered system provides detailed and accurate tracking of various aspects of websites, making it an essential tool for anyone who needs to stay informed about web page updates. The platform’s user-friendly design ensures that it is accessible to a broad audience, regardless of their technical background.
69. XML Sitemaps
XML sitemaps make it easier for search engines to crawl all your website’s pages and blog content. Creating and submitting sitemaps helps ensure all articles get properly indexed so readers can discover them.
Popular free plugins like Google XML Sitemaps for WordPress automate sitemap creation as you publish new posts. This makes it a simple optimization to boost organic search visibility every blogger should leverage.
70. Hotjar
Understanding how readers truly interact with your blog uncovers key areas for improvement. Hotjar reveals visitor behavior through session recordings, heatmaps, conversion funnels, form analysis, and polls to gather feedback.
Seeing website activity visually accelerates the identification of usability issues, debugging checkout processes, or enhancing page layouts/messaging to match reader expectations. Hotjar gives every blogger fly-on-the-wall access to the user experience.
71. Reddit
Long-form blog content resonates tremendously across interest-based subreddits, hungry for expertise around niche topics. Reddit is massively influential in directing mainstream conversations, so targeting specific communities introduces blogs to perfectly aligned new readers.
However, ensure content provides genuine value before participating. Rules vary widely between groups, so respectfully understand cultures beforehand. Embrace Reddit’s best practices, ultimately rewarding bloggers through referral traffic, branding, and backlinks.
72. YouTube Studio
YouTube Studio provides creators with a unified dashboard managing multi-channel analytics, video performance, comments, live streaming, merchandising, content planning, and more. Transitioning channels toward watch time and subscribers means optimization opportunities abound.
Reviewing comparative analytics for titles, descriptions, and thumbnails consistently improves future video clickability. Playlists boost authority and viewership by organizing content and surfacing related videos. Basics like captioning expand reach exponentially, too, while studio simplifies it all.
73. Buy Me a Coffee
Buy Me a Coffee offers simple yet effective blog monetization through one-time tips or recurring monthly pledges of reader appreciation in exchange for exclusive content. Connect instantly to global payment options.
Emphasizing community funding helps bloggers offset costs to keep content freely accessible to more readers. Video shoutouts for top supporters build loyalty, too. Custom stickers and homepage badges spread awareness, encouraging overall modest participation. Maintain creative freedom sustainably via Buy Me a Coffee.
74. Content Tools by Oberlo
Oberlo gained immense success, empowering e-commerce entrepreneurs to source inventory for resale online efficiently. Their new Content Tools aim at similar simplify-and-scale capabilities for bloggers around creating articles with enhanced automation.
Leveraging Oberlo’s extensive product catalog to get suggestions for trending commercial concepts could inform educational blog posts for respective audiences. AI-generated outlines and long-form drafts give a head start, saving tons of research and writing time. Publish faster at scale with Oberlo.
75. Buzzstream
Rekindling relationships and consistently pitching relevant ideas to past content partners maintains guest posting and backlink channels without intensive cold outreach demands. Buzzstream facilitates rediscovering and managing these relationships at scale.
Database management across outreach campaigns keeps tracking, reminders, and performance history centralized. Saved email templates also eliminate redundant communication tasks that quickly become administrative bottlenecks, especially at growth stages, with expanding collaboration rosters to nurture. Streamline networking with Buzzstream.
76. NetPeak Software
NetpeakSoftware is a platform offering specialized SEO software and tools aimed at enhancing website analysis and optimization. It caters to SEO specialists, webmasters, and anyone involved in website management and digital marketing. Here’s a detailed breakdown of what Netpeak Software provides:
- Advanced SEO Tools and Website Analyzer: The flagship product of Netpeak Software is the Netpeak Spider, an advanced website analyzer tool. Available for both Windows and macOS, this tool is designed to check over 100 technical issues on websites. It’s an essential resource for SEO professionals and webmasters, enabling them to conduct thorough website audits. The Netpeak Spider examines various aspects of a site, including but not limited to on-page SEO elements, link structure, and HTML tags. It helps identify SEO issues that could hinder a website’s performance in search engine rankings. Additionally, the tool offers data filtering and exporting options, making it easier to analyze and share insights.
- User-Friendly Interface and Effective SEO Task Management: Netpeak Software is known for its user-friendly interface, making complex SEO tasks more manageable and accessible even for those who are not deeply technical. The tools are designed to solve day-to-day SEO tasks in an easy and effective way. This includes optimizing on-page elements, tracking and analyzing backlinks, and monitoring overall website health. The software is tailored to meet the needs of both seasoned SEO professionals and those new to the field, providing a balance between advanced functionality and ease of use.
- Community and Support: Beyond its software offerings, Netpeak Software also fosters a community of users and provides robust support. This community aspect is beneficial for users looking to stay updated with the latest SEO trends and best practices. The company’s commitment to customer support ensures that users can get the most out of their tools, with guidance and assistance readily available.
In summary, NetpeakSoftware offers a suite of SEO tools and software primarily centered around the Netpeak Spider for website analysis. These tools are designed to identify and resolve a wide range of SEO issues, making them invaluable for website optimization. The platform combines advanced functionality with a user-friendly interface, catering to both expert SEO professionals and those new to the field.
77. Google Site Kit
Google Site Kit plugins streamline accessing analytical insights within WordPress, assisting content optimization for site owners. Popular modules include Page Speed Insights, Google Analytics dashboard, AdSense tracking, Site Search integration, and more in one convenient hub.
Understand audience acquisition channels better with visualized acquisition reports. Review page speed recommendations applying improvements for faster mobile experiences. Monitor income through AdSense dashboards. Enhance discoverability via Site Search adoption. Site Kit demystifies crucial capabilities for bloggers through thoughtful consolidation and presentation.
78. Amazon Alexa Skills
Alexa Skills enables bloggers and experts to be featured across Alexa-enabled devices like Amazon Echo as trusted resources from which users actively seek guidance on relevant topics. Position your brand as forward-thinking early adopters embracing voice search.
Consider predetermined frequently asked questions to answer or introduce fun mini experiences through interactive Alexa Skill ideas delighting fans. While adoption remains relatively niche today, gaining first-mover advantage secures your blog’s authority for when voice assistants inevitably mature into mainstream preference.
79. SerpStat
Serpstat is a comprehensive SEO management platform that offers a range of tools for search engine optimization (SEO), pay-per-click (PPC) advertising, and content marketing. It is designed to cater to the needs of digital marketers, SEO professionals, content creators, and businesses looking to enhance their online presence. Here’s a detailed breakdown of what Serpstat provides:
- Diverse SEO and Marketing Tools: Serpstat’s primary offerings include a suite of tools for backlinks analysis, rank tracking, keyword research, and competitor analysis. These tools are essential for understanding and improving a website’s performance in search engine results. The backlinks analysis tool helps in assessing the quality and quantity of backlinks pointing to a website, which is crucial for SEO. The rank tracking tool monitors a website’s search engine rankings for specific keywords, providing insights into its visibility. Keyword research tools are used to identify effective keywords for SEO and PPC campaigns, while competitor analysis tools help in understanding the strategies used by competitors and identifying opportunities for improvement.
- User-Friendly Interface and Comprehensive Data: Serpstat is known for its user-friendly interface, making it accessible for both SEO beginners and experts. The platform provides comprehensive data and analytics, allowing users to gain deep insights into their website’s SEO performance and the competitive landscape. This data-driven approach helps in making informed decisions to optimize websites for better search engine rankings and online visibility.
- Integration and Versatility: The platform also offers integration capabilities, such as a browser extension for instant SEO analysis of websites. This feature enhances the versatility of Serpstat, making it a convenient tool for quick SEO checks and in-depth analysis. Whether it’s for on-the-go analysis or detailed strategy planning, Serpstat provides a range of functionalities to suit various needs in the digital marketing space.
In summary, Serpstat is a versatile SEO management platform offering a wide array of tools for backlinks analysis, rank tracking, keyword research, and competitor analysis. Its user-friendly interface and comprehensive data make it suitable for a variety of users, from digital marketing novices to seasoned SEO professionals. The platform’s integration capabilities further add to its convenience and effectiveness in the realm of digital marketing and search optimization.
80. SiteChecker
Sitechecker is a robust SEO analysis and audit tool designed to help website owners, digital marketers, and SEO professionals improve their website’s search engine performance. The platform offers a range of features to analyze and optimize websites for better search engine rankings. Here’s a detailed breakdown of what Sitechecker provides:
- Comprehensive SEO Audit and Performance Data: The cornerstone of Sitechecker is its ability to perform detailed SEO audits. The platform consolidates organic search performance data from sources like Google Analytics and Google Search Console, along with its own analytical tools. This integration provides a holistic view of a website’s SEO health. The Site Audit tool, in particular, scans websites for common SEO issues, providing insights into aspects such as page speed, on-page SEO elements, and technical SEO factors. This comprehensive analysis helps website owners and SEO professionals identify and rectify issues that could be impacting their site’s search engine rankings.
- User-Friendly Interface and Real-Time Analysis: Sitechecker is designed with a user-friendly interface, making it accessible for users with varying levels of SEO expertise. The platform offers real-time analysis, allowing users to see the impact of their changes almost immediately. This feature is particularly useful for quickly testing and implementing SEO strategies. Additionally, the platform includes a Chrome Extension, which provides a convenient way to conduct on-page SEO checks directly from the browser. This extension helps users quickly identify and fix technical SEO issues, further improving their website’s ranking potential.
- Versatile SEO Tools and Resources: Beyond audits and real-time analysis, Sitechecker offers a range of other SEO tools and resources. These include backlink tracking, keyword tracking, and competitor analysis, providing users with comprehensive tools to manage various aspects of their SEO strategy. The platform also offers educational resources and guidance, making it a valuable tool for both learning and implementing SEO best practices.
In summary, Sitechecker is a versatile and user-friendly SEO analysis and audit platform. It offers a comprehensive suite of tools for auditing, real-time analysis, and ongoing SEO management, making it a valuable resource for anyone looking to improve their website’s search engine performance. Whether you’re a seasoned SEO professional or a website owner starting to explore SEO, Sitechecker provides the tools and insights needed to succeed in the competitive world of search engine optimization.
81. Feedly
Feedly stays ahead as the leading RSS feed reader, aggregating updates from bloggers and publications into one convenient app. One-click adding optimizes scanning headlines daily across fixed customized sources. Recommendations also improve discovering new potentially relevant follows.
Stay on the pulse of industry news, trending stories, or niche topics monitoring real-time Feedly stream. Keyword and author alerts notify timely about priority content moments after emerging, too. Maintain focus while still intellectually exploring relevant peripheral perspectives through Feedly.
82. Sprout Social
SproutSocial is a comprehensive social media management platform that offers a range of tools and solutions for businesses and organizations to manage their social media presence effectively. The platform is designed to cater to the needs of various users, from small businesses to large enterprises, looking to harness the full potential of social media for their marketing and communication strategies. Here’s a detailed breakdown of what Sprout Social provides:
- All-in-One Social Media Management: Sprout Social’s primary offering is its all-in-one social media management solution. This includes tools for publishing and scheduling social media posts, managing and responding to social media interactions, and monitoring social media channels. The platform supports a variety of social media networks, allowing users to manage multiple accounts from a single interface. This centralized approach makes it easier for businesses to maintain a consistent and active social media presence across different platforms.
- Analytics and Reporting: A key feature of Sprout Social is its robust analytics and reporting capabilities. The platform provides detailed insights into social media performance, including metrics on engagement, audience growth, and content effectiveness. These analytics help businesses understand their social media impact and make data-driven decisions to optimize their strategies. Additionally, Sprout Social offers tools for listening and tracking social conversations, enabling businesses to stay informed about relevant topics and trends in their industry.
- User-Friendly Interface and Collaboration Tools: Sprout Social is known for its user-friendly interface, which is designed to be accessible to users of all skill levels. The platform also offers collaboration tools, making it easy for teams to work together on social media management tasks. This includes features for assigning tasks, sharing drafts, and approving content, which is particularly useful for larger teams or agencies managing social media for multiple clients.
In summary, SproutSocial offers a powerful and versatile social media management solution that encompasses publishing, engagement, analytics, and collaboration. Its comprehensive suite of tools is designed to help businesses of all sizes unlock the full potential of social media, transforming their online presence and audience engagement. With its user-friendly interface and robust analytics, Sprout Social is a valuable asset for anyone looking to enhance their social media strategy.
83. Medium Memberships
Medium pioneered long-form writing, attracting 194 million monthly users and high search visibility. Their newly launched Membership offering establishes recurring income streams for creators directly proportional to loyal support built around niche thought leadership.
Fans subscribe, unlocking exclusive content while funding writers fairly. Bloggers may consider migrating wholly into building audiences inside the Medium ecosystem given established reach plus a built-in monetization model upon proving expertise – essentially securing your blog income sans ads.
84. SocialPilot
SocialPilot manages organic social media publishing across Facebook, Instagram, Twitter, LinkedIn, and Pinterest, synchronizing campaign management simplified for solopreneurs juggling responsibilities solo otherwise. Collaborating staff additionally brainstorm and schedule content collectively.
Custom analytics measure engagement, too. Approval workflows ensure branding consistency and minimize mistakes before public visibility. Consolidate social procedures via a user-friendly SocialPilot dashboard perfect for bloggers lacking dedicated marketing resources behind owned platforms. Sync calendar integrations with Google Calendar or Office 365.

85. WeVideo
WeVideo is an online video editing platform that offers a user-friendly and accessible solution for creating and editing videos. Designed for beginners and professionals alike, WeVideo provides a range of features and tools to make video editing simple and efficient. Here’s a detailed breakdown of what WeVideo offers:
- Easy-to-Use Video Editing Tools: WeVideo’s main attraction is its online video editor, tailored for users of all skill levels, from beginners to more experienced creators. The platform allows users to capture, create, view, and share videos easily. It supports editing videos in resolutions up to 4K, ensuring high-quality output for various purposes. Users can access various editing tools, including cutting and trimming video clips, adding text and transitions, and incorporating music and sound effects. The drag-and-drop interface simplifies the editing process, making it accessible even for those without video editing experience.
- Collaboration and Accessibility: WeVideo is designed to facilitate collaboration, allowing multiple users to work on a project simultaneously. This feature is particularly useful for teams, educators, and businesses that require collaborative video projects. Additionally, being a cloud-based platform, WeVideo enables users to edit videos from any device with internet access, offering flexibility and convenience. Users can start editing on one device and continue on another, making it a versatile tool for on-the-go editing.
- Creative Resources and Sharing Capabilities: The platform also offers a library of creative resources, including stock video clips, images, and music tracks, which users can incorporate into their projects. This vast collection of resources enhances the creative possibilities for users. Once a video is completed, WeVideo provides easy sharing options, allowing users to publish their videos directly to social media platforms or download them for offline use.
In summary, WeVideo is a comprehensive online video editing platform combining ease of use and powerful editing capabilities. It is suitable for many users, from individuals looking to create personal videos to professionals and educators working on collaborative projects. With its cloud-based nature, extensive resource library, and flexible sharing options, WeVideo stands out as a convenient and versatile video creation and editing tool.
86. Strikingly
Strikingly website builders require zero coding skills to create 100% mobile-optimized sites and landing pages essential for bloggers capturing subscriber signups and selling products like eBooks or online course seats. Great conversion and load speeds improve appeal.
Add popular elements like slideshows, countdowns, testimonials, and calls to action freely. Easy custom domain connectivity plus built-in SEO strengths enhance discoverability on Google Search and Maps. Blog successfully with Strikingly speed and performance advantages.
87. Circleboom
Circleboom empowers creators to monetize niche audiences by offering community membership capabilities, exclusive blog content, and digital goods for paid annual or monthly subscribers. Custom packages deliver personalized experiences.
Foster deeper reader engagement via community rewards or behind-the-scenes content promises, converting one-time blog visitors into recurring brand supporters. Member forums and profile badges cultivate exclusivity, too. Professional templates accelerate setup without engineering or design demands.
88. RightBlogger
RightBlogger is an AI-powered content creation suite made specifically for bloggers. It’s the ultimate toolbox I wish I had when first starting out. With over 50 creation and productivity tools, RightBlogger makes writing high-quality, SEO-friendly content easy.
By combining the latest AI advancements with an intuitive interface, RightBlogger empowers bloggers to craft engaging articles, headlines, social posts, and more in seconds. The platform adds new tools weekly based on user feedback, giving subscribers direct input into new features.
89. Social Dog
SocialDog is an all-in-one marketing tool for Twitter and Instagram that offers automation, analytics, and account management services. It is designed to help you streamline your operations and become more efficient while increasing your engagement. The tool is used by over one million users, including individuals and companies from various industries.
With SocialDog, you can manage your Twitter and Instagram accounts with ease. The tool provides post management, account analytics, and follower management features. You can craft your posts in advance and schedule them to be posted at a specified time. You can even schedule posts in bulk to save time. SocialDog also helps you improve engagement and communicate efficiently with your followers. You can monitor keywords and hashtags, track competitors, and analyze your account’s performance.
SocialDog offers four plans for individuals and companies to choose from according to their Twitter and Instagram operations. The plans are priced reasonably and offer a full range of features necessary for social media marketing. SocialDog is the perfect tool for your Twitter and Instagram account management needs.
90. Squirrly Products
Squirrly is a digital marketing platform offering various tools and services to help entrepreneurs and businesses improve their online presence. The platform provides AI-powered SEO tools, social media management, and content marketing solutions.
With Squirrly, you can optimize your website’s SEO with ease. The platform offers an all-in-one SEO suite that takes the guesswork out of search engine optimization. It provides over 650 pre-configured features during installation, making managing and improving your site’s SEO easy. Squirrly also offers a range of social media management tools that help you schedule and publish posts, monitor keywords and hashtags, and track your competitors.
Squirrly offers a variety of plans to suit different needs and budgets. The plans are priced reasonably and offer a full range of features necessary for digital marketing. Squirrly is the perfect tool for entrepreneurs and businesses looking to improve their online presence and reach more customers.
91. EZ Mob
EZmob is a global advertising network offering various solutions for website owners, publishers, advertisers, agencies, and app developers. The network provides monetization for display and mobile ads, as well as access to over 1 billion users across the world.
With EZmob, you can choose from different mobile ad formats, traffic sources, and platforms to suit your needs and goals. You can use the self-serve client interface to create and manage your campaigns or work with the managed accounts team to get expert support. EZmob also offers various tools and features to help you optimize your performance and reach more customers.
EZmob offers four plans for users: publishers, advertisers, agencies, and app developers. The plans are priced competitively and offer various benefits and features. EZmob is the perfect network for your online advertising needs.
92. WP Forms
WPForms is a user-friendly WordPress plugin allowing you to create and manage online forms easily. The plugin offers a range of features, such as a drag-and-drop form builder, pre-built templates, and smart workflows to help you save time and effort.
With WPForms, you can create contact forms, online surveys, donation forms, order forms, and other WordPress forms in just a few minutes without writing any code. The plugin is 100% responsive and works on mobile, tablets, and desktops. WPForms also provides smart conditional logic to create high-performance forms and instant notifications to respond to leads quickly.
WPForms offers a range of plans to suit different needs and budgets. The plans are priced reasonably and offer a full range of features necessary for online form creation. WPForms is the perfect tool for entrepreneurs and businesses looking to create smart online forms easily.
93. SignNow
SignNow is an e-signature solution that allows you to sign, send, and manage documents online with legally binding e-signatures. The platform offers a range of features, such as smart workflows, document management, and enterprise-grade security and compliance.
With SignNow, you can sign documents online by typing, drawing, or uploading your signature. You can also save your signature for future use or to sign additional documents. The platform provides an all-in-one solution for document management, including features such as document templates, document sharing, and document storage.
SignNow offers a range of plans to suit different needs and budgets. The plans are priced reasonably and offer a full range of features necessary for online document management. SignNow is the perfect tool for entrepreneurs and businesses looking to streamline their document management processes and improve their workflow.

94. Tidio
Tidio is a customer service platform offering various tools and features to help businesses improve their customer support and communication. The platform provides an all-in-one solution for live chat, chatbots, ticketing, and multichannel communication.
With Tidio, you can automate your customer service and boost conversion rates with the help of AI-powered chatbots. The platform offers over 35 pre-built chatbot templates to help you engage users and sell more. You can also use the visual chat builder to create custom chatbots without any coding skills. Tidio also provides live chat and ticketing features to help you manage your customer support efficiently.
Tidio offers a range of plans to suit different needs and budgets. The plans are priced reasonably and offer a full range of features necessary for customer service and communication. Tidio is the perfect tool for improving customer support and client communication.

95. Writer.com
The writer leverages AI to automatically generate SEO blog posts through intuitive UX, specifying key elements like target keywords, tone of voice, and other parameters for computer authorship. Request various formats as needed, tailoring output to reuse and repurposing initial creation across channels.
Limited free monthly word count allows trialing quality before paid monthly subscriptions fit advanced blogger demands. Combining human strategy with machine creation production, sustainable workflows emerge natively, building blogs with infinitely scalable capacities, futureproofing success over relying solely on manual efforts longer-term, or hiring proportional writing teams with impractical small operators.
96. Blogshare
Blogshare symbolizes win-win relationships between bloggers seeking visibility and brands desiring genuine expertise advocating modern consumers through valuable thought leadership editorially. Mutually beneficial partnerships between independent publishers and marketers are fostered when content resonates.
Amplify your blog’s subject authority and storytelling, converting relevant brand awareness into recurring readership. Maintain editorial independence while financially sustaining content operations at any scale. Blogshare welcomes all genres and categories as brands increasingly value genuine creator partnerships overtly.
97. Socialinsider
Accelerate social media growth 10X through Socialinsider’s analytics dashboards, revealing engaging user-generated content opportunities, optimal posting times, competitor tactics, and network-specific recommendations tailored to respective category trends. Onboard privately managed accounts securely.
Review historical performance of tagged content informing smarter hashtags, mentions, and @ strategies built upon collective learnings. Benchmark metrics to industry standards, highlighting gaps needing priority attention next. Let social media senior expertise guide bloggers clinching next-level growth through Socialinsider.
98. Ayrshare
Ayrshare offers APIs that make it easier to use social media platforms like Facebook, Twitter, and LinkedIn from other websites and apps. For example, Ayrshare has to post APIs that let you schedule social media content and automatically publish posts to your accounts on these platforms without logging in. You can also use Ayrshare’s analytics API to get simple reports on how well your content is performing across all your connected social accounts.
In simpler terms, the Ayrshare social APIs act as helpers that take information from apps and websites and share it to your social media pages. The APIs also collect data on how many people saw or interacted with those posts. So you don’t have to visit all your actual social media accounts to update, post, or check performance. You can view simple reports or get notified about your social media results right within the app or website you’re working on. Using Ayrshare social APIs makes managing multiple social media accounts much easier!
99. Eleven Labs
ElevenLabs is a text-to-speech and voice cloning software that lets you create and use lifelike voices in any language and style. The platform offers over 1300 ultra-realistic voices covering different languages and dialects, ensuring that you can create videos with voiceovers in your desired language with ease. You can also use it as an online text reader, a voice generator, a voice library, and a voice assistant. The platform is ideal for creating educational videos, explainers, product demos, social media content, YouTube videos, TikTok Reels, and video ads.
With ElevenLabs, you can generate high-quality spoken audio in any voice, style, and language. The AI voice generator is powered by an AI model that renders human intonation and inflections with unrivaled fidelity, adjusting the delivery based on context. You can clone your voice or create synthetic voices in minutes and instantly convert text to speech in any language. You can also personalize your AI voice with a choice from a diverse range of more than 2,000 human-sounding voices in over 75 languages. The text-to-video tool is fully web-based, so you only need a device with internet access and a browser to create, edit, and publish your videos. You can export your videos in formats like MP4. ElevenLabs also offers a free tier that allows users to explore text-to-voice and text-to-video features without any cost. You can generate 5 minutes of free audio and video content per month. However, certain advanced features and premium AI capabilities may require a paid subscription.
ElevenLabs is SOC 2 compliant, an industry standard for data protection, and built with your privacy and protection in mind. You can trust ElevenLabs with your valuable data.
100. ADCopy
AdCopy is an AI-based tool that helps businesses create high-quality advertisement copy that resonates with their target audience. The platform offers a range of features that allow you to create professional ad copy without the need for expensive equipment, inconsistent actors, complex editing tools, or third-party teams. With AdCopy, you can generate high-converting ad copy quickly and easily, even if you don’t have a background in marketing or copywriting. The AI-powered copy is designed to grab attention and drive action. You’ll get ad copies optimized for various platforms and campaigns, saving you hours of writing and revisions.
AdCopy is ideal for businesses looking to create engaging and effective ad copy for their products or services. You can use AdCopy to produce compelling ad copy for your social media campaigns, Google Ads, Facebook Ads, and more. The platform offers a range of ad copy templates that you can customize to suit your brand’s voice and style. You can also use AdCopy to generate ad copy for your landing pages, email campaigns, and other marketing materials. AdCopy’s AI-powered copy is designed to be persuasive and engaging, helping you drive conversions and grow your business.
AdCopy is SOC 2 compliant, an industry standard for data protection, and built with your privacy and protection in mind. You can trust AdCopy with your valuable data. AdCopy also has an ethical approach to AI, emphasizing acceptable use and transparency.
101. Beehiiv
Beehiiv is a newsletter platform that offers a range of features to help you create, edit, publish, and monetize newsletters and websites. The platform is built for growth and is ideal for creators, publishers, and companies looking to scale and monetize their audiences. Beehiiv was created by a few early employees at Morning Brew, who witnessed firsthand the power and influence of having a large and engaged audience.
With Beehiiv, you can access the best tools available in email, helping your newsletter scale and monetize like never before. The platform offers a range of ad copy templates that you can customize to suit your brand’s voice and style. You can also use Beehiiv to generate ad copy for your landing pages, email campaigns, and other marketing materials. Beehiiv’s AI-powered copy is designed to be persuasive and engaging, helping you drive conversions and grow your business.
Beehiiv is SOC 2 compliant, an industry standard for data protection, and built with your privacy and protection in mind. You can trust Beehiiv with your valuable data. Beehiiv also has an ethical approach to AI, emphasizing acceptable use and transparency.
In summary, Beehiiv is a powerful newsletter platform that offers a range of features to help you create, edit, publish, and monetize newsletters and websites. Whether you want to start a new media brand, grow your existing one, or scale faster, Beehiiv has the features and support you need.
Conclusion
The blogging landscape moves incredibly fast, with new platforms and capabilities launching constantly. I urge bloggers to continually reevaluate their stacks against the latest tools and features in 2024, which are covered here.
Maximizing your site’s reach and revenue requires keeping pace with readers’ needs and expectations. Failing to advance your toolkit risks leaving potential and flexibility on the table during this competitive, noisy era for digital publishers.
Hopefully, walking through the most indispensable updates gives all skill levels ideas for enhancing productivity and insight. Please ask any questions on implementing these tools for your blog specifically. And if you would like to see more lists of tools like this one, please let me know in the comments and what sort of list you would like to see next.
Artificial Intelligence
A Comprehensive Guide to the Best AI Writing Tools: Expert Recommendations.
This article provides a comprehensive overview of the top 10 AI writing tools as voted by their peers. The tools include Grammarly, Writesonic, Quillbot, Jasper, OpenAI GPT-3, Wordtune, ContentBot, Article Forge, Hemingway, and ProWritingAid. Each tool has its own unique features and pricing options. The article concludes by stating that the choice of the best AI writing tool ultimately boils down to personal preferences, budget, and UI design.
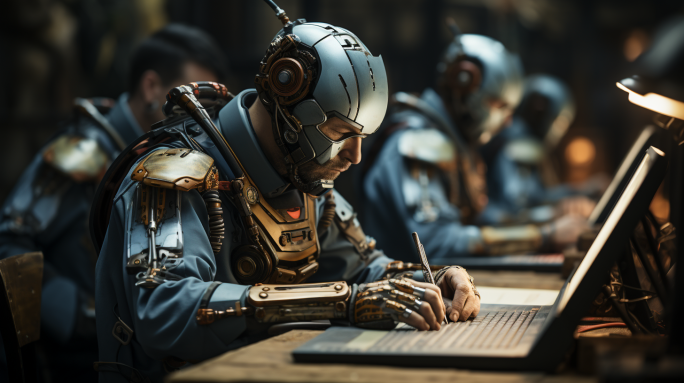
In a quest to identify the best AI writing tools available, an innovative approach was taken: asking 39 AI writing tools to recommend their top 10. The results provide a fascinating insight into the AI writing tool landscape, showcasing the most favored tools by AI themselves. Here, we present the top 10 AI writing tools as voted by their peers, along with key features and pricing details.
Unveiling the Top AI Writing Tools
1. Grammarly (67% of votes)
Pricing: Free; paid plans from $10/month
Grammarly, primarily known as a typing assistant, has expanded its capabilities with AI text generation features (GrammarlyGO) introduced in April 2023. This tool assists in content creation and reviews it for grammar, spelling, punctuation, and clarity. A notable feature is its built-in plagiarism checker, which is beneficial given the tendency of large language models (LLMs) to plagiarize content unintentionally.
2. Writesonic (62% of votes)
Pricing: Free up to 10,000 words/month; paid plans from $20/month
Writesonic serves as an AI-powered writing assistant, facilitating the creation of various content types, including ad copy, text messages, product descriptions, and more. Its Brand Voice feature ensures consistency and tone with AI-generated content, offering a tailored approach to content creation.
3. Quillbot (56% of votes)
Pricing: Free; paid plans from $19.95/month
Quillbot offers a multifaceted platform with features for paraphrasing, grammar checking, plagiarism detection, and summarization. Its ability to generate new ideas, examples, and viewpoints makes it a versatile tool for content generation.
4. Jasper (54% of votes)
Pricing: Paid plans from $49/month
Jasper, formerly known as Conversion.ai and Jarvis, stands out for its marketing-focused capabilities. It offers templates for popular copywriting frameworks and a “Ridiculous Marketing Ideas” feature, providing creative sparks for unique marketing concepts.
5. OpenAI GPT-3 (49% of votes)
Pricing: Free; $20/month for Plus
GPT-3, the precursor to the widely used ChatGPT, remains a versatile tool for all writing-related tasks. Despite being based on training data up to 2021, it’s still a reliable tool for creating outlines, blog posts, and other content types.
6. Wordtune (46% of votes)
Pricing: Free (10 rewrites and three prompts/day); paid plans from $9.99/month
Originally a rewriter, Wordtune now also generates content. Its rewriting feature suggests multiple variations for any highlighted sentence or paragraph, and its “Spices” prompts enhance content with unique elements like historical facts or jokes.
7. ContentBot (41% of votes)
Pricing: $1 for Prepaid (free 10K words); paid plans from $19/month
ContentBot is another AI-powered tool aiding in the quick generation of high-quality content. It covers various content types and offers a “Prompts” library for template selection. A highlight is its ability to generate entire landing pages using just keywords.
8. Article Forge (38% of votes)
Pricing: $27-$247/month (depending on the number of words)
Like other tools on this list, Article Forge speeds up content generation. Unique features include specifying topics to exclude, adding images and videos, and replacing keywords with links.
9. Hemingway (36% of votes)
Pricing: Free (web); one-time payment of $19.99 for the app
Hemingway, primarily a content readability grader, is rumored to be adding AI rewriting features. While not an AI writing tool per se, it helps improve the clarity and readability of content.
10. ProWritingAid (33% of votes)
Pricing: Free (500-word limit); $30/month for premium OR one-time payment of $399
ProWritingAid, known for its grammar-checking capabilities, also offers rephrasing suggestions. It’s more akin to Grammarly than AI content generators like Jasper, but it still plays a crucial role in refining content.
Other Plugins, Services, and Tools that may interest you. These are affiliate links some I use, some I don’t, so before using, please investigate thoroughly SemRush, Invideo, Squirly Products like Squirly SEO, Gravity Forms, Wpforms, OptinMonster, Buy Website Traffic, AI-powered Marketing, Press Release Distribution Made Easy, Track and Protect your Online Marketing, #1 Press Release Distribution Service, Email lists and Distribution Tech, Placeit Envato, Monster Insights, SmashBallon, Icegram, HubSpot, WPwebHost, ConvertKit, Rytr, Canva, QuillBot, Shortly AI, Market Muse, Content Studio, Social Pilot, Strinkingly, CircleBoom, TailWind, Hotjar, The Hoth, Linkilo, CleanMail, HexoWatch, NetPeak, SerpStat, SiteChecker, SproutSocial, WeVideo, Social Dog, EzMob, Sign Now, Tidio, WP Data Tables, Copymatic, HeyGen, Synthesia, Fliki, ElevenLabs, ADcopy, Beehiiv, EduBirdie.
Final Thoughts
This list, perhaps not surprisingly, showcases the most recognized and trusted tools in the AI writing domain. Based on similar technology and often limited by their training data, these tools still provide a valuable benchmark for those seeking the best AI writing tools. Ultimately, the choice boils down to personal preferences, budget, and UI design, making this list a comprehensive guide for anyone looking to enhance their content creation process with AI.
Artificial Intelligence
Streamlining Content Marketing with AI: Strategies and Cutting-Edge Tools
AI is transforming content marketing by automating mundane tasks, offering predictive analytics, and facilitating more creative and impactful storytelling. AI addresses several content marketing challenges, including the dependency on key personnel for thought leadership content. By leveraging AI tools like Writer.com, ChatGPT, and Claude.ai, marketers can summarize complex topics, streamline the research process, and enhance content creation and repurposing. Tools like Jasper.ai, Gloss.ai, Munch, and Opus Clip can turn blog posts or articles into engaging social media content and segment long-form videos into captivating promotional clips, respectively. AI in content marketing not only boosts efficiency but also ensures that your content resonates with your audience, giving you a competitive edge in the ever-evolving digital landscape.
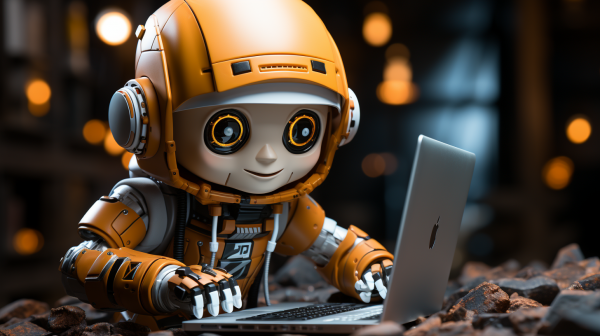
In today’s digital era, where content marketing plays a pivotal role in business success, leveraging AI tools can significantly streamline and enhance your content creation process. As AI continues to revolutionize content production, understanding how to effectively utilize these tools is key for any marketer looking to stay ahead of the curve. This article delves into how AI can optimize your content marketing workflows, providing insights and practical tips.
The Importance of AI in Content Marketing
AI is transforming content marketing by automating mundane tasks, offering predictive analytics, and facilitating more creative and impactful storytelling. It enables marketers to produce diverse content types such as text, images, and audio at scale, and tailor them to specific audience preferences.
Overcoming Content Marketing Challenges with AI
AI addresses several content marketing challenges, including the dependency on key personnel for thought leadership content. For example, the Marketing AI Institute restructured its podcast process, allowing for more efficient content creation and distribution by leveraging AI. This strategic shift not only saved time but also enhanced the quality and relevance of their podcast.
Repurposing Content Effectively
AI also plays a crucial role in maximizing the utility of existing content by identifying opportunities for repurposing. It helps marketers avoid the pitfalls of creating unrelated content that doesn’t align with their marketing goals.
Developing a Robust Content Marketing Strategy with AI
While AI significantly boosts efficiency, human expertise remains essential in enriching AI-generated content with unique insights. The future of content creation lies in combining AI’s capabilities with human creativity to produce distinctive and engaging content.
Expedite Content Preparation with AI
AI tools like Writer.com, ChatGPT, and Claude.ai can summarize complex topics, making them accessible to your audience. Generative AI platforms, such as Perplexity AI, offer concise descriptions of search results, streamlining the research process for content creators.
Enhancing Content Creation and Repurposing
Tools like Descript assist in producing professional-level audio and video content, enabling efficient repurposing of core content like podcasts into articles, social media posts, and more.
Streamlining Social Media Posts
AI tools such as Jasper.ai help in maintaining a consistent presence on social media platforms, turning blog posts or articles into engaging social media content, thereby alleviating the cognitive load of content creation.
Short-form video Creation with AI
In the realm of short-form video, AI tools like Gloss.ai, Munch, and Opus Clip can segment long-form videos into captivating promotional clips, saving time and enhancing social media engagement.
Conclusion
AI is redefining content marketing by offering tools that simplify and enhance the content creation process. From streamlining research to automating social media posts, AI empowers marketers to focus on creative storytelling while managing the technical aspects of content production. Embracing AI in content marketing not only boosts efficiency but also ensures that your content resonates with your audience, giving you a competitive edge in the ever-evolving digital landscape.
-
Tips & Tricks4 months ago
WordPress Security Hacks
-
Pages4 weeks ago
Write For Us – Guest Post
-
Showcase1 month ago
StylizedWeb.com
-
News3 weeks ago
How to: Show/Hide any div box with jQuery in WordPress
-
Tips & Tricks3 months ago
How to: show/hide a widget in WordPress with jQuery
-
Tips & Tricks19 hours ago
Remove the title attribute using jQuery
-
Plugins2 months ago
Top Membership plugins
-
Tips & Tricks1 month ago
Limit the characters that display on the_title
You must be logged in to post a comment Login